Actionable Evidence
Unlocking Real-Time Evidence for Practitioners
Q&A with Erika Van Buren, First Place for Youth and Peter York, BCT Partners
Moderated by Kelly Fitzsimmons, Project Evident
As part of Project Evident’s Actionable Evidence Initiative, our Founder and Chief Executive Kelly Fitzsimmons recently sat down with First Place for Youth’s Chief Innovation Officer Erika Van Buren and BCT Partners’ Principal Peter York for a webinar to discuss the use of data science and machine learning to provide First Place for Youth program staff with actionable evidence they could use on demand. The piece below represents highlights from the webinar. The full Actionable Evidence case study on First Place for Youth and BCT Partners is available here.
Pete York
BCT Partners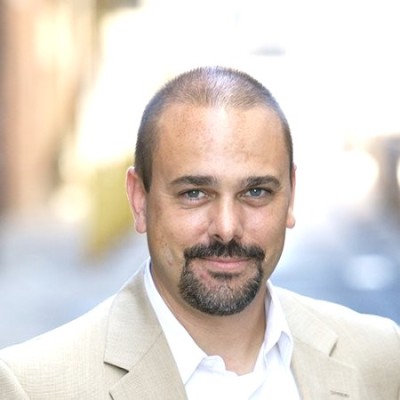
Erika Van Buren, Ph.D.
First Place for Youth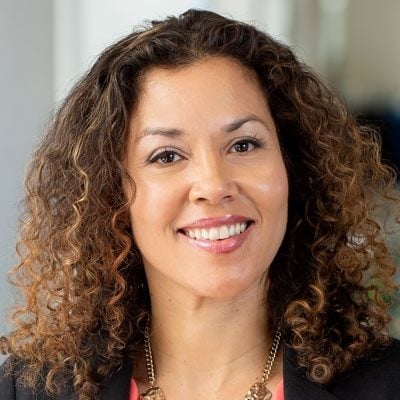
Kelly Fitzsimmons
Project Evident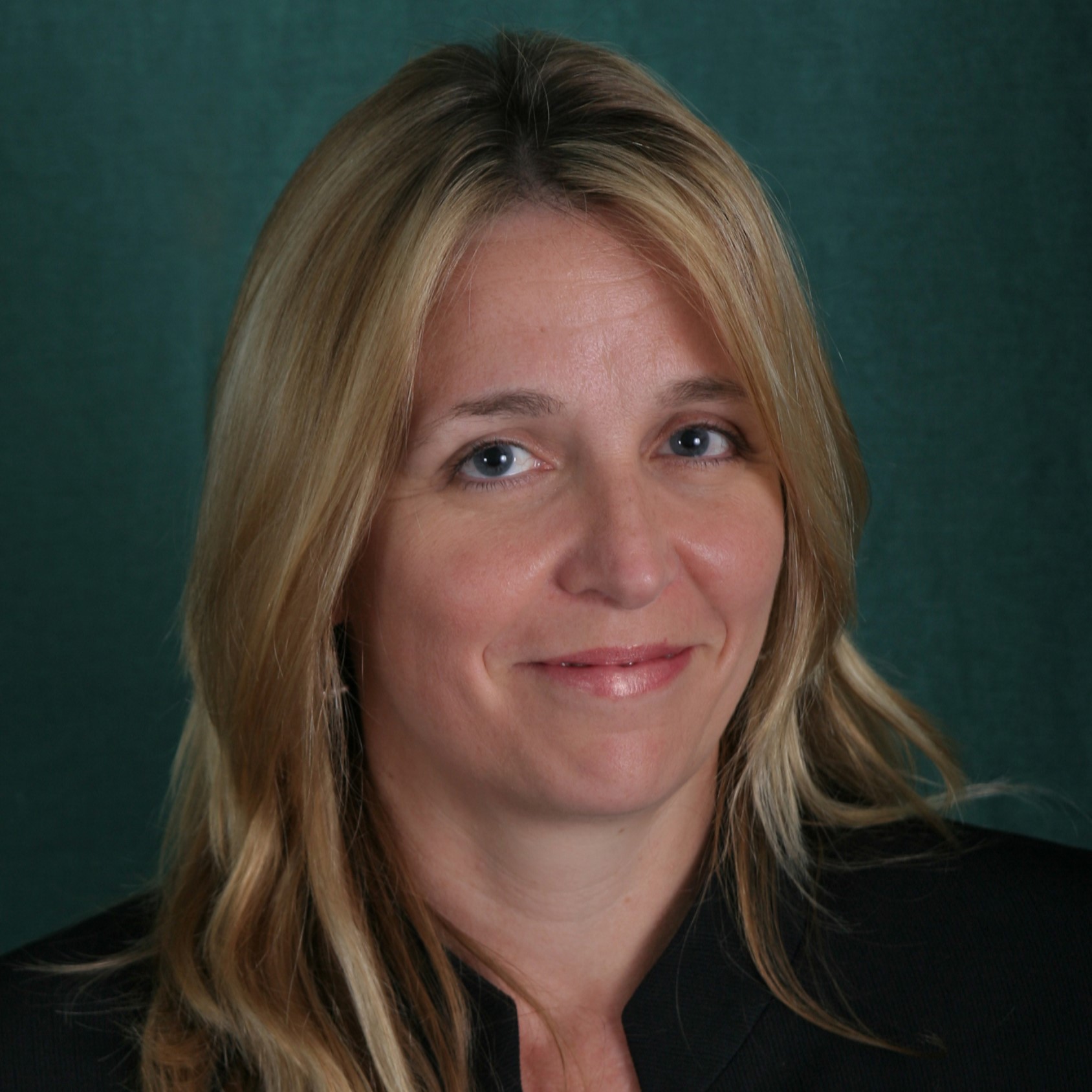
KELLY FITZSIMMONS: Tell us a little bit about First Place for Youth (FPFY) and BCT Partners, and why FPFY started this journey of evidence building with BCT Partners?
ERIKA VAN BUREN: FPFY is both a direct service and an advocacy organization. We provide a stable foundation of housing, employment, and education services to young people who have either aged out of the foster care system or have opted into extended foster care. We have a core program model, called the My First Place program model, where our direct services work is centralized. What makes FPFY unique is that we not only use data knowledge as a primary program learning and improvement tool, but we demonstratively leverage data as a program intervention itself. As an organization, we have always invested in addressing core and continuous learning questions — who are we serving? Who is more or less likely to engage in our services in a way that sets them up for success?
“Evidence would guide both policy and funding decisions, so it had immense value, but it wasn’t reaching the people who were doing the work every day. “
Pete York, BCT Partners
To answer these questions, we collect rich program data, but we wanted to apply our data to support our practitioners’ strategic learning in real time. That is what led us to work with BCT Partners back in 2014, to develop what became known as the Youth Roadmap to Success tool. This tool modeled all our administrative data as the backbone for a decision support tool that provides recommendations for practice and gauges progress with our core result of interest: young people earning lifelong living wage employment.
PETE YORK: A bit about my background — I am a former social worker and frontline caseworker for the homeless, and I became an evaluator after graduate school. The more I learned about evidence, the more I realized it wasn’t reaching practitioners who were on the ground. Evidence would guide both policy and funding decisions, so it had immense value, but it wasn’t reaching the people who were doing the work every day.
The FPFY and BCT partnership was anchored in a practitioner-centric, youth-first approach. FPFY had already collected data and wanted to get to the next evidence level, and BCT Partners had done a lot of work in both foster care and juvenile justice, so we could understand the nuance of the complexity of a pivotal transition for children aging out of foster care.
At BCT partners, we had begun to integrate predictive analytics tools, machine learning and Artificial Intelligence (AI) to enhance program administrative tools. These tools are of critical importance to the social sector. We see it all the time in the for-profit and data sector, you are watching Netflix and it can recommend the next movie you should watch or you are on Amazon and it can recommend the next book you should read, based on previous ones you’ve finished. Usually, if I take those recommendations, they are effective. So the question is – how can we bring these machine learning and data science tools to bridge the gap between evidence and the population base to what the practitioner needs in real time?
KELLY: Machine learning in the social sector is new. When we think about algorithms, we tend to think about bias, which is of particular importance as we’re dealing with human subjects. Can you speak to some of the concerns that arose as you were embarking on this initiative?
“I knew nothing about data science before this project, and I want to normalize that for people.”
Erika Van Buren, First Place for Youth
ERIKA: I knew nothing about data science before this project, and I want to normalize that for people. I had no idea how it worked. I knew it was something that was used at Google, and in my mind it was big data. I was concerned if we would have enough data to do this. Will this be meaningful? From a traditional evaluation standpoint, power is determined by sample sizes. I wasn’t convinced we had enough data to do this, until I talked to Pete.
PETE: People often ask, ‘aren’t the models changing all the time?’ Not necessarily. Change doesn’t happen all at once. So as new data comes in, we have to make adjustments and retrain the models.
“People forget that data is reflective of the people making and documenting decisions and those decisions have been filtered through their own social and individual biases.”
Pete York
Bias is something we have to be watchful of. If we run algorithms for predictive accuracy only, we will perpetuate both research and social biases. People forget that data is reflective of the people making and documenting decisions and those decisions have been filtered through their own social and individual biases. As an organization, BCT Partners is very intentional about this. If race or gender shouldn’t be the reason someone does or does not get a program, then we don’t select based on that. This ensures each group is equally getting what works so we have a way to assess equity. I can go into more detail but transparency is key, not using algorithms that no one can see what is going on inside. If you want to put science after the word “data” then peer review and transparency has to be there. So that we’re not in the business of black box algorithms and just dumping every variable in there to get the highest accuracy. It has to have explanatory power and not perpetuate biases.
KELLY: Can you explain more about the tool FPFY and BCT Partners developed? How automated was it?
ERIKA: We use Apricot for our performance management system and every day it shoots to our data warehouse and then it goes through the models. I’m doing a very elementary explanation of this so forgive me. It then goes to our business intelligence software Power BI which is connected to our SharePoint site. People can go into our portal and easily access this information. The data doesn’t live outside of the existing technical architecture for the organization which is a huge win for us.
KELLY: How did your initial thinking and design evolve over time and over the course of the project? What did you learn about that and what capacities did FPFY and your stakeholders build?
ERIKA: We’ve had two generations of this tool and the second one is far superior, in part because we developed a logic model with our data to guide the modeling and that has made it incredibly relevant to the way we work. That is how we get people to enter the data, they’re entering it because they’re maintaining fidelity to our model, they’re documenting outcomes for young people and that data is looked at all the time already. It was a game changer for us because now people can connect the dots between what we do with incremental, individualized and close to real-time outcomes.
KELLY: Pete, can you clarify your definition of “natural experiments”?
PETE: As many nonprofit organizations know, evaluation reports have a shelf life because the data is not continuously updated. With data science and computer engineering, it is. Using those tools you can take data that is already being collected and leverage it.
A real world example – when I was a caseworker for the homeless, some people would get referred to substance abuse treatment and some would not because there weren’t enough beds available. That is a natural experiment. You have a counterfactual (referred to treatment vs. not referred to treatment) and now you can study those two cases and all similar cases and match based on their backgrounds. Because the real world doesn’t always present the opportunity to have those combinations of treatments in the same dosages, those are natural experiments. Machine learning makes it possible to study those patterns of differences, not just one variable at a time but as a combination and as they interact.
Working with FPFY, we looked back historically and saw treatment variation within each FPFY group and we leveraged those differences to identify patterns of what works.
KELLY: How do you get funders to see the work differently and understand the value of these approaches and this type of evidence building?
ERIKA: Getting a basic skills job is not enough and we have to be able to support young people to be self-sufficient. That became our strategic directive, and this has been fully embedded into the way we think about our mission. We explain this to our funders, who love this by the way. It helps with explaining what our mission is and being demonstrative about this is one of the tools we have to elevate practice-based evidence. This is practice-based evidence at its finest. We use it as a tool to help them understand the complexity of the work – what does it take to achieve an outcome that is long lasting and endurable and makes a real difference in a young person’s life?
KELLY: I’m curious when you look at the project to date, which aspects of the Actionable Evidence Framework have you played out in the past, and where do you anticipate they may play out in the future as you are building your capacity in this work?
ERIKA: This tool was built with a lens on equity. We incorporated what we learned into a strategy for eliminating and addressing racial and ethnic disparities. We did this while building practitioner capacity, which to me was one of the biggest wins throughout the whole process.
A year ago we launched an apprenticeship model that was more robust and rigorous, and we are about to develop a tool to identify the type of education to employment, to get young people living wage employment. Everyone in the program is using the youth roadmap tool to test different elements of the apprenticeship model over time.
PETE: If we really want to get to that place of true equity, how do we bring in more community voices and youth voices interacting with the tool itself? So that it’s their tool and not just for practitioners. The Actionable Evidence Framework resonates so well because it’s meeting at the space where the practitioner meets the youth, and I think if we can get to the youth, we’ll have it rounded out.
KELLY: Erika, how did the dashboard help you see the change in decisions your team made once they had access to this new data? How has the quality of the decision making shifted or changed? Has there been any tension between what the data is saying and what case managers say based on their own judgment as practitioners?
Erika: This is not a tool that replaces your judgment as a practitioner; it is an additional tool in your arsenal. It is really intended to expand the range of resources available to practitioners to pick and choose from, and the practitioners tell us how they want to be able to use it.
“We have to be transparent because that is where the power of a practitioner’s experience and wisdom comes in and there is no way the data is going to give us all of that.”
Pete York
PETE: At BCT Partners, we have this philosophy that the outputs from the models are just probabilities. That is, we want the practitioner to understand that what the model is really saying is that in 60% of cases with this history we have a successful outcome and in 40% we don’t, so that the model isn’t making the decision. At some point, you have to ensure buy-in from practitioners so that you empower people to make the final decision (not the algorithm).
So we have to be transparent because that is where the power of a practitioner’s experience and wisdom comes in and there is no way the data is going to give us all of that.
KELLY: How do you maintain data quality throughout this process? Can you speak to organizations that are smaller or have limited data and aren’t sure where to even begin?
PETE: Before beginning the precision modeling process, a data audit is required to evaluate the quality and quantity of data to determine “readiness.” Based on prior program administration modeling projects, BCT Partners has determined that the following minimum data qualities are required: (1) a minimum of 250 cases to allow for the disaggregation of cases into matched comparison groups, (2) at least two years of reliable longitudinal intake, program, and outcome data; (3) low levels of missing data; and (4) high levels of variability for key questions/ measures. Additionally, both organizations requested additional preliminary analyses to test the feasibility of generating results during the full precision modeling process.
When BCT Partners worked with FPFY, there were challenges at each of these stages. One of the most salient for many organizations is low-quality program intervention data. Many program datasets do not capture enough structured data on the qualitative experience to provide rich explanatory insights. These data don’t typically offer much, if any, information about the modalities (e.g. cognitive behavior therapy), methods (e.g. 1:1 therapeutic session), and/or breakdowns of how time was spent. The best solution for this program was to make data improvements that would benefit future precision modeling updates by integrating stronger questions into the administrative data collection system.
KELLY: As you reflect on your journey in this work, what were some of the challenges you faced?
ERIKA: When California began implementing extended foster care, it shifted First Place for Youth’s work from being outside the system to being a provider inside the system. This affected who came into our program and their unique needs and types of experiences, and our current program was not adequately addressing what their needs were. We had collected all this data on a program model that only worked for a subset of our population, and didn’t necessarily reflect where we were trying to go. I was increasingly concerned that our program modeling wouldn’t reflect the current landscape of service delivery for organization.
We were fortunate that Pete involved program people in the creation of the algorithm from the beginning. It was so important that there were other perspectives to interpret the data and elucidate the spurious associations and the associations that could actually be harmful for the young people we work with.
PETE: Let’s say there is a big system event. You were outside of the system (e.g. California made sweeping changes to service delivery) that affected the population you serve. As the new data comes in, we have to make adjustments and retrain the models. It’s like in criminal justice reform when a state legalizes marijuana, and you’re building models to understand justice factors. People ask, ‘aren’t the models changing all the time?’ Not necessarily because all of these changes don’t happen immediately.
Both policy changes and system changes can affect what we are learning in the data when we do the modeling. I think that is also where there is a readiness, that has to be there that understands where the opportunity is. You can evolve your models and really learn from that.
The full Actionable Evidence case study is available here.
Generating On-Demand, Actionable Evidence: First Place for Youth and Gemma Services
Read the case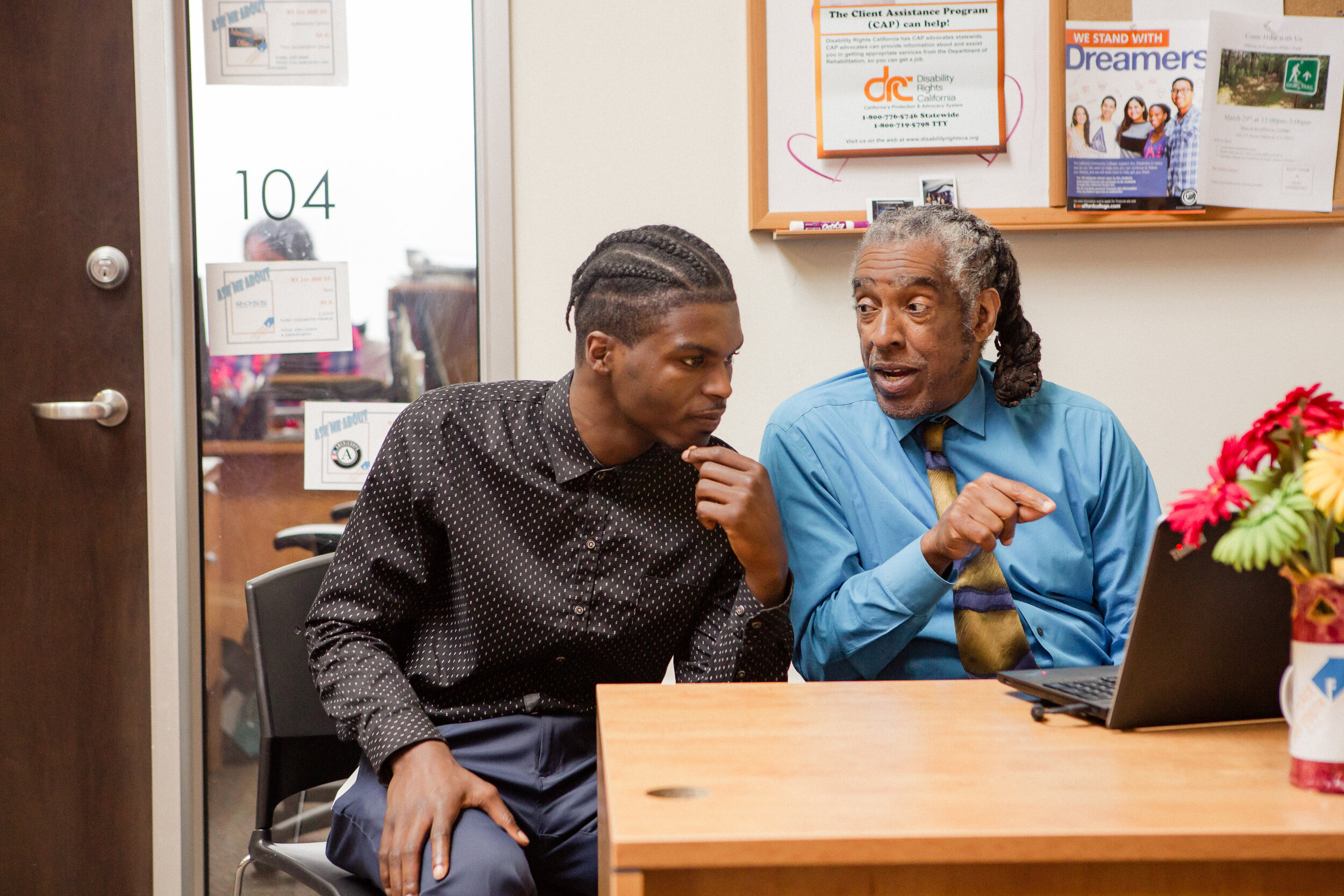